I-O Biomarkers: Discovering the Possibilities
Research in the field of Immuno-Oncology (I-O) biomarkers seeks to characterize the relationship between the immune system, the tumor and its microenvironment, and the host. Unique interactions of these factors, as well as I-O biomarker presence and prevalence, contributes to the balance of activation versus suppression of the antitumor immune response.1-3
Tumors can be characterized based on their degree of immune-cell infiltration, ranging from noninflamed to inflamed.4 I-O biomarkers that can identify inflamed tumors may help predict a pre-existing antitumor immune response.3,5 Therefore, the goal of I-O biomarker testing is to help enable a more personalized approach to treatment by identifying patients who are likely to respond to specific immunotherapies.3,5,6
I-O biomarkers can be classified into the following categories: tumor antigens, inflamed tumor markers, immune suppression markers, and host environment factors.5,7,8
Bristol-Myers Squibb is committed to the exploration of biomarkers in I-O research.
Biomarkers in Immuno-Oncology Research
Biomarkers can help guide clinical decisions
Biomarkers are biologic molecules, cells, or processes found in tissues or body fluids (such as blood) that are a sign of a normal or abnormal process or disease.1,2 The goal of biomarker testing is to individualize cancer treatment by targeting the right patients with the right therapy at the right time. Three common types of biomarkers include:
- Prognostic biomarkers may identify the likelihood of a clinical event, such as disease progression, disease recurrence, or death, independent of the therapy received.3,4 For example, the expression level of a protein on tumor cells may be associated with poor disease outcome independent of treatment
- Predictive biomarkers are present at diagnosis and may identify whether individuals are more likely to experience a favorable or unfavorable response to treatment.3,4 For example, the presence or upregulation of a protein on tumor cells may correlate with a favorable outcome in response to a certain treatment
- Pharmacodynamic biomarkers may show that a biologic response has occurred in an individual who has received treatment.4,5 For example, comparing tissue- or blood-based assay results before, during, and afte treatment may reveal changes in the level of a protein, indicating that the therapy has had a biologic effect
I-O biomarkers can be indicators of antitumor immune activity
I-O biomarkers are a type of biomarker that can help evaluate an active antitumor immune response within the body.6
While the immune system seeks to detect and destroy tumor cells, the tumor attempts to evade or suppress immune activity. The balance between antitumor immune activation and suppression results from complex interactions among several factors, including:7-9
- The interplay between tumor and immune cells within the tumor microenvironment10
- Tumors can be characterized based on their degree of immune-cell infiltration, ranging from noninflamed to inflamed11
- The host environment, which can modulate antitumor immune activity12
The field of I-O biomarkers aims to characterize these ongoing interactions.13
I-O biomarkers are a dynamic and diverse subset of biomakers
Descriptions of I-O biomarkers and traditional genetic driver mutations represent common features of each group, but are not exhaustive
I-O biomarkers are distinct from traditional genetic driver mutation biomarkers. While I-O biomarkers measure dynamic immune activity within the tumor microenvironment and host environment, genetic driver biomarkers measure stable DNA alterations within a tumor.9 For example, traditional genetic driver mutations such as EGFR and BRAF tend to have binary expression patterns that are either present or absent.9,14-17 They are typically homogenously expressed within a tumor.18
In contrast, I-O biomarkers are not binary and have a range of expression or magnitude.19-21 Heterogeneous within the tumor microenvironment, they are dynamic in nature and can be inducible.12,22-24 I-O biomarkers can also vary based on the stage of disease and location.22,23,25,26
Bristol-Myers Squibb is committed to researching I-O biomarkers in the following categories:
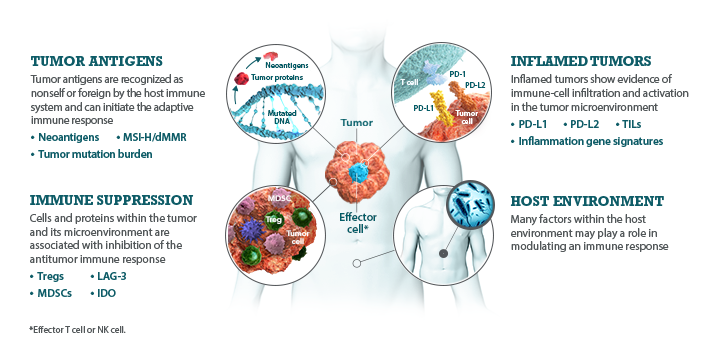
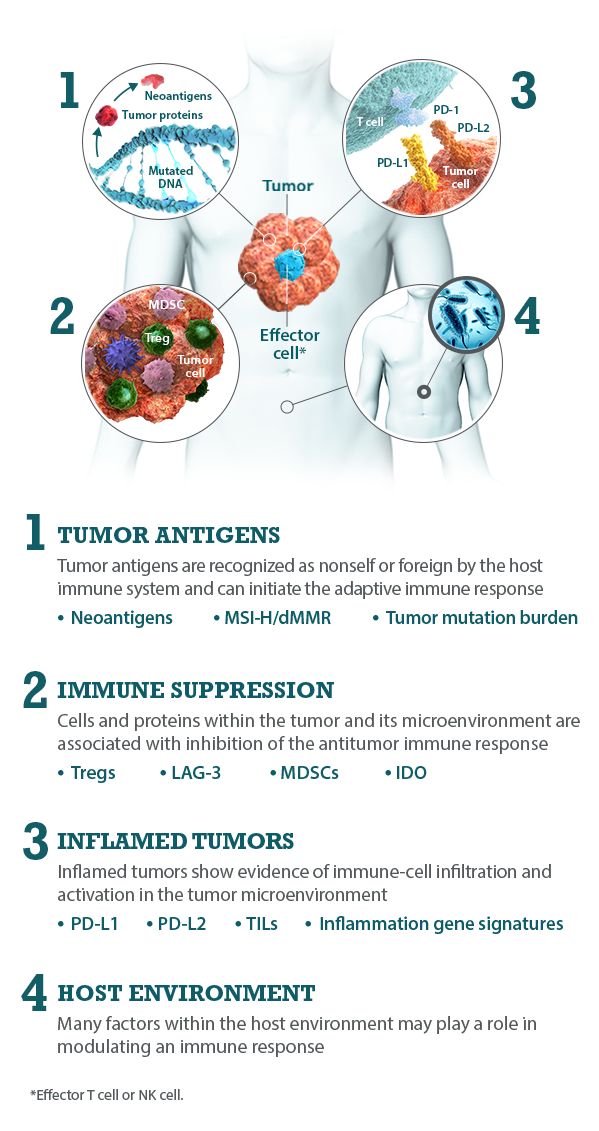
Multiple I-O biomarkers may be needed to provide a more precise representation of the tumor microenvironment
As components and regulators of the immune response, I-O biomarkers are dynamic and complex.8,9 The immune response is regulated by an intricate network of activating and inhibitory signaling pathways.27,28 Therefore, the presence or absence of any single I-O biomarker may not provide a complete understanding of the diverse interactions occurring within the tumor microenvironment.9,29 Evaluating multiple I-O biomarkers in combination may provide a more accurate and comprehensive assessment of immune status.9
Learn more about categories of I-O biomarkers
REFERENCES: Discovering the Possibilities of I-O Biomarkers
1. Gkretsi V, Stylianou A, Papageorgis P, Polydorou C, Stylianopoulos T. Remodeling components of the tumor microenvironment to enhance cancer therapy. Front Oncol. 2015;5:214. 2. Nelson D, Fisher S, Robinson B. The ‘‘trojan horse’’ approach to tumor immunotherapy: targeting the tumor microenvironment. J Immunol Res. 2014. doi:10.1155/2014/789069. 3. Sharma P, Allison JP. The future of immune checkpoint therapy. Science. 2015;348(6230):56-61. 4. Hegde PS, Karanikas V, Evers S, et al. The where, the when, and the how of immune monitoring for cancer immunotherapies in the era of checkpoint inhibition. Clin Cancer Res. 2016;22(8):1865-1874.
REFERENCES: Biomarkers in Immuno-Oncology Research
1. Henry NL, Hayes DF. Cancer biomarkers. Mol Oncol. 2012;6(2):140-146. 2. Strimbu K, Tavel JA. What are biomarkers? Curr Opin HIV AIDS. 2010;5(6):463-466. 3. Ballman KV. Biomarker: predictive or prognostic? J Clin Oncol. 2015;33(33):3968-3971. 4. US Food and Drug Administration. About biomarkers. www.fda.gov/Drugs/DevelopmentApprovalProcess/DrugDevelopmentToolsQualificationProgram/BiomarkerQualificationProgram/ucm535922.htm. Accessed August 1, 2017. 5. Gainor JF, Longo DL, Chabner BA. Pharmacodynamic biomarkers: falling short of the mark? Clin Cancer Res. 2014;20(10):2587-2594. 6. Whiteside TL. Immune responses to cancer: are they potential biomarkers of prognosis? Front Oncol. 2013;3:107. 7. Gkretsi V, Stylianou A, Papageorgis P, Polydorou C, Stylianopoulos T. Remodeling components of the tumor microenvironment to enhance cancer therapy. Front Oncol. 2015;5:214. 8. Nelson D, Fisher S, Robinson B. The ‘‘trojan horse’’ approach to tumor immunotherapy: targeting the tumor microenvironment. J Immunol Res. 2014. doi:10.1155/2014/789069. 9. Sharma P, Allison JP. The future of immune checkpoint therapy. Science. 2015;348(6230):56-61. 10. Balkwill FR, Capasso M, Hagemann T. The tumor microenvironment at a glance. J Cell Sci. 2012;125(Pt 23):5591-5596. 11. Hegde PS, Karanikas V, Evers S, et al. The where, the when, and the how of immune monitoring for cancer immunotherapies in the era of checkpoint inhibition. Clin Cancer Res. 2016;22(8):1865-1874. 12. Chen DS, Mellman I. Elements of cancer immunity and the cancer-immune set point. Nature. 2017;541(7637):321-330. 13. Yuan J, Hegde PS, Clynes R, et al. Novel technologies and emerging biomarkers for personalized cancer immunotherapy. J Immunother Cancer. 2016;4:3. 14. Davies H, Bignell GR, Cox C, et al. Mutations of the BRAF gene in human cancer. Nature. 2002;417(6892):949-954.